Unlocking the Future: Top 5 Books for Mastering Machine Learning
Welcome to our blog on the interesting world of AI. Machine learning has become incredibly important in today’s technological landscape, which is moving at a rapid pace. The whole idea is to teach computers to learn and grow through experience without being explicitly programmed. Cool, correct?
In the age of Google and online tutorials, you might be wondering why we’re talking about learning from books. Let me tell you, authoritative books on machine learning are extremely valuable. They provide a solid foundation and an in-depth comprehension of the topic that goes beyond merely scratching the surface.
AI isn’t simply some extravagant idea limited to the domains of the scholarly world. It is actively reshaping industries all over the place. The impact of machine learning is undeniable in every sector, from entertainment to transportation to finance to healthcare. It’s upsetting the manner in which we decide, computerize cycles, and concentrate bits of knowledge from huge measures of information.
Thus, go along with us as we plunge into the universe of AI and find how books can be your confided-in colleagues in this elating experience. Prepare to gain access to a wealth of information and the abilities that will shape the future. Let’s get started…
“Pattern Recognition and Machine Learning” by Christopher Bishop
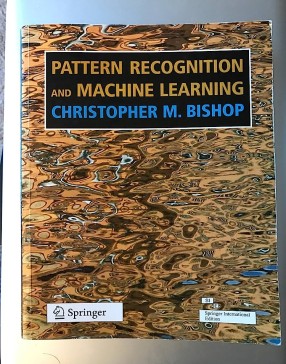
Christopher Bishop’s brilliant book “Pattern Recognition and Machine Learning” is the first book of our blog. In the field of machine learning, Bishop is a well-known figure, and his book has become a go-to resource for experts and beginners.
A. Key Concepts and Topics Covered in the Book:
- Statistical Learning: Bishop provides readers with a solid foundation in statistical learning by instructing them on how to decode complex data patterns and derive useful insights.
- Bayesian Methods: A comprehensive understanding of feasibility modelling and its application to machine learning issues is provided in the book.
- Design Recognition Techniques: By examining a variety of methods for pattern recognition, Bishop provides readers with the knowledge necessary to recognize patterns in data and make accurate predictions.
- Supervised and Unsupervised Learning: The book explains how to train models using labelled data and how to find hidden patterns in unlabeled data, covering both supervised and unsupervised learning methods.
- Deep Learning and Neural Networks: Bishop investigates the entrancing universe of brain organizations and profoundly gets the hang of them, giving experiences into their designs and how they can be used for tackling complex issues.
B. Practical Applications and Real-World Examples:
- Medical Diagnostics
- Natural Language Processing
- Financial Analysis
- Image and Speech Recognition
These are only a couple of glimpses into the practical applications and real-world examples discussed in Bishop’s book.
“Deep Learning” by Ian Goodfellow
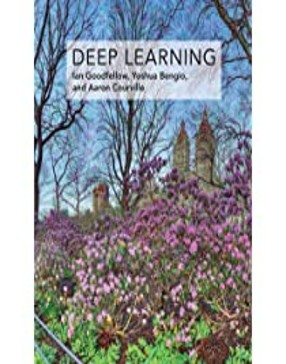
“Deep Learning,” written by Ian Goodfellow, a talented author. A well-known figure in the field of machine learning, Goodfellow’s book has become widely regarded as an important resource for understanding the depths of deep learning.
A. Deep Learning’s Importance in Machine Learning:
Unleashing the Power of Neural Networks: Neural networks are elevated to a whole new level by deep learning. It makes it possible to build deep neural networks with multiple layers, which allows the models to learn and represent complex data patterns.
Dealing with Big Data: Deep learning is especially strong with regard to taking care of large-scale datasets. It can really extract important data from vast amounts of data, revealing complicated connections and secret examples that could somehow or another slip through the cracks.
Exceptional Performance: Deep learning has reliably accomplished cutting-edge execution in different areas, for example, PC vision, natural language processing, and speech recognition. By pushing the limits of what machines can do, it has changed these fields.
- Computer vision tasks made possible by deep learning include accurate image recognition, object detection, image segmentation, and even the generation of realistic images.
- In NLP, deep learning models have made significant progress, making it possible to perform tasks like sentiment analysis, language translation, text generation, and systems that respond to questions.
- By assisting in the analysis of medical images, diagnosis of diseases, personalized treatment recommendations, and drug discovery, deep learning is revolutionizing healthcare.
- Deep learning plays an important part in autonomous vehicle technology, empowering object discovery, path recognition, and, surprisingly, self-driving capabilities.
B. Comprehensive Coverage of Deep Learning Techniques in the Book:
These points simply start to scratch the extensive coverage you’ll track down in Goodfellow’s book.
- Neural Network Architectures
- Training Deep Networks
- Deep Learning Applications
- Theoretical Foundations
“Machine Learning: A Probabilistic Perspective” by Kevin P. Murphy
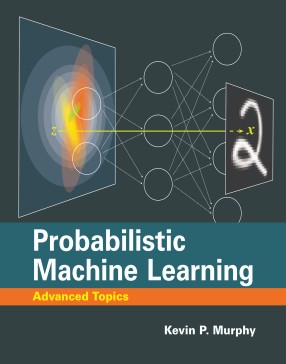
Another fascinating book is “Machine Learning: A Probabilistic Perspective” by Kevin P. Murphy. Murphy has made significant contributions to the field of machine learning, and his book has received widespread praise.
A. Key Topics Covered in the Book:
Let’s now look at some of the most important topics covered in Murphy’s book. The following are a couple of significant focuses to consider:
- Graphical Models: The concept of graphical models, which offer a visual representation of feasibility relationships between variables, is the main focus of the book. Understanding complex dependencies and making predictions based on evidence is made easier with this framework.
- Bayesian Systems: Murphy explores Bayesian networks, a type of graphical model that makes use of Bayesian inference to model uncertainty and predict probabilities. These networks are especially helpful while dealing with data that is uncertain and incomplete,
- Probabilistic Graphical Models: The book covers probabilistic graphical models, which combine both probabilistic and graphical modelling techniques. They are essential in many machine learning applications because they are effective representations and powerful tools for uncertainty.
B. Comprehensive Coverage and Balance between Theory and Practice:
Theoretical Concepts: The book provides a solid theoretical foundation for probabilistic machine learning by providing concise and understandable explanations of key concepts, principles, and mathematical formulations.
Application in Practice: Murphy emphasizes the practical application of probabilistic machine learning methods alongside the theory. Case studies, code examples, and helpful advice for using these methods in real-world situations are all included in the book.
Integration of Various Perspectives: In order to present a comprehensive perspective on probabilistic machine learning, Murphy’s book seamlessly integrates concepts from a variety of fields, including statistics, computer science, and artificial intelligence.
Now, let’s turn our attention to the real-world uses of probabilistic machine learning that Murphy discusses in his book. The following are a few features:
- Natural Language Processing
- Recommender Systems
- Bioinformatics
- Financial Modeling
“The Hundred-Page Machine Learning Book” by Andriy Burkov
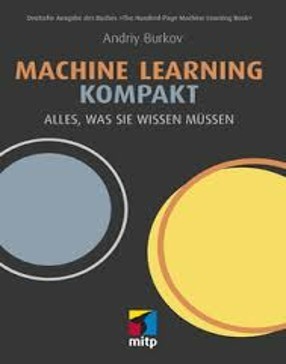
By condensing the vast field of machine learning into just one hundred pages, Burkov accomplished something truly remarkable with this “The Hundred-Page Machine Learning Book” book. It serves as a concise but powerful introduction to the fundamentals of machine learning.
A. A Standout Method for Condensing Machine Learning Ideas:
“The Hundred-Page Machine Learning Book” By condensing complex machine learning concepts into a concise format, Burkov takes a novel approach. Consider these few points:
Distilling the Essentials: The book cuts through the noise and explains the fundamentals of machine learning in a way that is easy to understand. It quickly introduces you to the fundamental concepts, like a concentrated shot of knowledge.
Concise and Accessible: By collecting the material into 100 pages, Burkov ensures that the content is effectively available to both experts and beginners with some limited time. To understand the main points, you don’t have to read a lot of text.
Streamlined Learning Path: Readers are taken step by step through the fundamental concepts in the book which provides a structured learning path. It covers key topics, algorithms, and strategies without overpowering the readers with unnecessary details.
B. Author’s Expertise in Simplifying Complex Concepts:
As he simplifies complex machine learning concepts without sacrificing depth, Andriy Burkov’s expertise shines through. Some examples of his expertise include:
- Burkov is skilled at carrying complex ideas in a clear and concise manner. He simplifies complex concepts into easily understandable content making it simpler for readers to understand the fundamentals.
- The author adds practical examples to the theoretical ideas to make sure that readers can put the ideas into practice in real-world situations. This reasonable method improves the opportunity for growth and overcomes any barrier between hypothesis and practice.
- Even with the book’s condensed format, Burkov ensures that readers deep understanding of the core concepts.
- He provides sufficient substance to foster a solid foundation in machine learning by striking a balance between simplicity and depth.
In this way, with “The Hundred-Page AI Book,” you can expect an extraordinary and efficient learning opportunity that enables you with the basics of AI in a short and accessible way.
“Machine Learning Yearning” by Andrew Ng

Our last peak from the Machine Learning book is “Machine Learning Yearning” by Andrew Ng. Andrew Ng is well-known for his significant contributions and expertise in the fields of machine learning and artificial intelligence. He is a respected authority in the field because he was a co-founder of Coursera and has been involved in groundbreaking research and industry projects.
A. Focus on Real-World Applications and Practical Aspects:
The focus on practical aspects of machine learning and the real-world application of algorithms is one of the fascinating aspects of “Machine Learning Yearning.” Consider these a few points:
Problems in the Real World: The book discusses real-world difficulties associated with applying machine learning to a variety of fields. It provides readers with assistance in navigating the practical aspects of implementation by delving into the variations and complexities that arise when deploying machine learning systems.
Algorithm Selection: Ng highlights the significance of selecting the appropriate algorithms for particular tasks. He discusses how to select appropriate algorithms based on the problem at hand, the available data, and the desired outcomes. So that readers understand the variation of algorithm selection by discussing
Systems for Machine Learning: The book explores the bigger image of AI frameworks and their mix into real-world situations. It provides readers with a comprehensive understanding of the practical aspects of building machine learning systems. By addressing important considerations like system design, scalability, and reliability.
B. Structure and Emphasis on Iterative Development and Data Quality:
These points give you a bit glimpse into the valuable experiences and useful direction you can expect from “Machine Learning Yearning.” Let’s discuss the structure and main points:
- Continuous Improvement: Ng accentuates the significance of iterative improvement in AI projects. He guides readers through an iterative process, encouraging them to incorporate feedback, evaluate performance, and iterate on the design to continuously improve their models.
- Importance of Data Quality: The book focuses a lot on the quality of the data. Ng features the basic job of information in AI projects and examines best practices for data collection, cleaning, and preprocessing. He helps readers to understand how to deal with problems related to data and explains how data quality affects model performance.
Conclusion
We have highlighted some outstanding books for mastering machine learning throughout our discussion. These books include Christopher Bishop’s “Pattern Recognition and Machine Learning,” Ian Goodfellow’s “Deep Learning,” and “Machine Learning: A Probabilistic Viewpoint” by Kevin P. Murphy, and “The Hundred-Page AI Book” by Andriy Burkov, which offer priceless information and bits of knowledge.
On your path to becoming an expert in machine learning, these books that have been recommended to you can serve as guidance. These books cover important ideas, real-world examples, and practical applications in detail. It gives you the ability to understand the complexity of machine learning and use it to solve difficult problems in a variety of fields.
Therefore, I recommend you read these books and begin your own learning journey. Take in the information on their pages and allow it to fuel your enthusiasm for machine learning. Keep in mind, becoming capable in this field is a continuous process, and these books will act as trusted companions along the way.
Also Read
The Power of Blockchain: Exploring the Best Books on Blockchain Technology